Data-Centric AI: The Future of Artificial Intelligence
Data-Centric AI. Unlike the traditional model-centric approach that emphasizes the architecture and algorithms, data-centric AI shifts the focus to the quality and management of data.
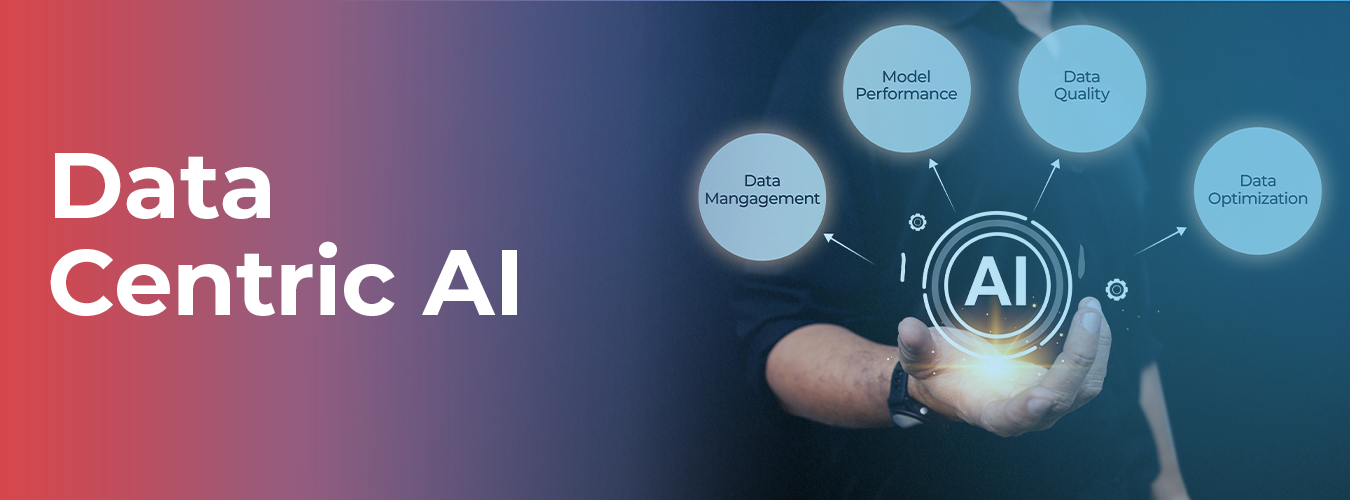
In the evolving landscape of Artificial Intelligence (AI), one approach has been gaining significant attention: Data-Centric AI. Unlike the traditional model-centric approach that emphasizes the architecture and algorithms, data-centric AI shifts the focus to the quality and management of data. This method is increasingly recognized as crucial for the advancement of AI technologies, providing a more robust foundation for building reliable and accurate AI models. In this blog, we will explore the importance of data-centric AI, its advantages, and how it’s shaping the future of AI development.
What is Data-Centric AI?
Data-centric AI is an approach where the primary focus is on the quality of data used to train AI models rather than solely on improving model architectures. The premise is simple: better data leads to better models. This approach involves curating, cleaning, and labeling data meticulously to ensure that the AI models learn from the best possible dataset. By refining the data, organizations can often achieve better results with simpler models, reducing the need for complex algorithms.
The Shift from Model-Centric to Data-Centric AI
Traditionally, AI research and development have been model-centric, where the emphasis is placed on developing more sophisticated algorithms and models. However, as AI applications become more widespread, the limitations of this approach are becoming apparent. Complex models require massive amounts of data, and the quality of that data significantly impacts the model’s performance.
Data-centric AI addresses these limitations by prioritizing the preparation and management of data. This shift is especially important in real-world applications where data is often noisy, incomplete, or biased. By focusing on data quality, AI practitioners can create models that are more generalizable and less prone to errors.
Benefits of Data-Centric AI
-
Improved Model Performance: High-quality data allows AI models to learn more effectively, leading to improved accuracy and performance. With well-curated data, even simple models can achieve impressive results.
-
Reduced Complexity: By focusing on data rather than complex algorithms, the development process becomes more straightforward. This reduction in complexity can save time and resources, making AI development more accessible.
-
Better Generalization: Data-centric AI helps in creating models that generalize better to new, unseen data. This is crucial in applications like autonomous driving or medical diagnostics, where the AI must perform reliably in diverse environments.
-
Enhanced Fairness and Bias Reduction: One of the critical challenges in AI is the presence of biases in training data, leading to unfair or discriminatory outcomes. Data-centric AI emphasizes the identification and mitigation of such biases, resulting in fairer models.
-
Scalability: As AI is applied to more industries and use cases, the need for scalable solutions is growing. Data-centric AI allows organizations to scale their AI efforts more effectively by streamlining the data management process.
Applications of Data-Centric AI
Data-centric AI is being adopted across various industries to enhance AI applications. Some of the prominent areas include:
-
Healthcare: In healthcare, the accuracy and reliability of AI models can be a matter of life and death. Data-centric AI ensures that medical AI systems are trained on high-quality, representative data, leading to more accurate diagnoses and treatments.
-
Autonomous Vehicles: Autonomous driving relies heavily on AI models that can make split-second decisions. Data-centric AI plays a crucial role in ensuring these models are trained on diverse and comprehensive datasets, improving safety and reliability.
-
Finance: In the finance sector, data-centric AI helps in creating models that can detect fraudulent activities, assess credit risk, and optimize trading strategies. The emphasis on data quality ensures that these models are robust and trustworthy.
-
Retail: Retailers use AI to personalize customer experiences, optimize supply chains, and forecast demand. By focusing on high-quality data, retailers can improve the accuracy of their AI models, leading to better business outcomes.
Challenges in Implementing Data-Centric AI
While data-centric AI offers many benefits, it also comes with challenges. One of the main obstacles is the time and effort required to curate and label data. Additionally, ensuring that data is representative and free from bias can be difficult, especially in large, complex datasets.
Another challenge is the need for collaboration between data scientists, domain experts, and data engineers. Data-centric AI requires a multidisciplinary approach to ensure that the data used in AI models is of the highest quality.
Conclusion
Data-centric AI is not just a trend but a fundamental shift in how we approach AI development. By prioritizing data quality over model complexity, organizations can build more reliable, fair, and scalable AI systems. As the field of AI continues to evolve, embracing a data-centric approach will be key to unlocking the full potential of AI and driving innovation across industries.
For those looking to stay ahead in the AI race, investing in data-centric AI practices will be crucial. By focusing on the quality of your data, you can create AI models that are not only more accurate but also more aligned with the diverse and dynamic nature of the real world.
What's Your Reaction?
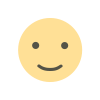
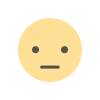
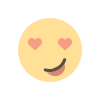
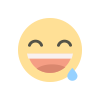
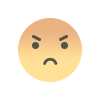
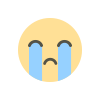
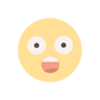