Integrating AI and Machine Learning in Pest and Disease Forecasting
The integration of Artificial Intelligence (AI) and Machine Learning (ML) in pest and disease forecasting offers a promising solution by providing precise, timely predictions and enabling more effective field management system
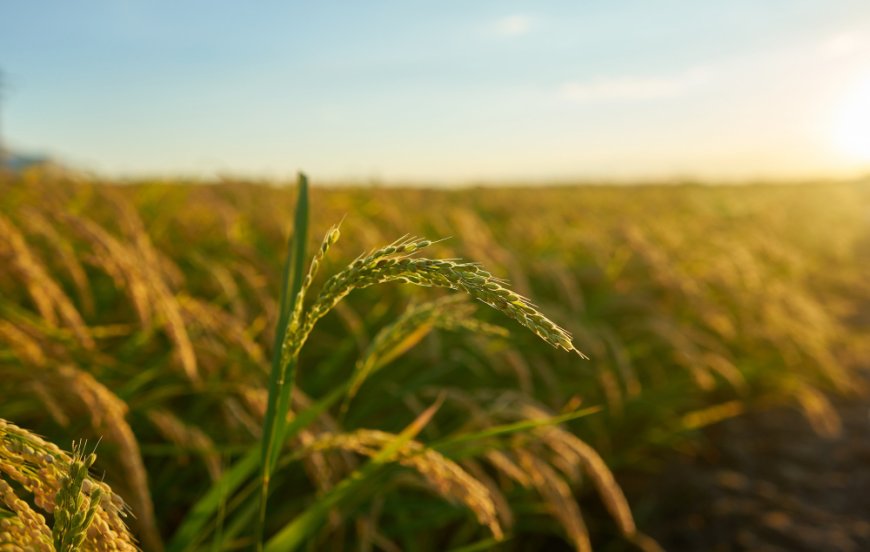
Pest and disease outbreaks pose significant threats to agricultural productivity, impacting both the quantity and quality of crops. Traditionally, pest management has relied on historical data, visual inspections, and chemical treatments. However, these methods can be reactive rather than proactive, often leading to substantial losses before control measures are implemented. The integration of Artificial Intelligence (AI) and Machine Learning (ML) in pest and disease forecasting offers a promising solution by providing precise, timely predictions and enabling more effective field management system.
The Role of AI and Machine Learning in Agriculture
AI and ML have revolutionized numerous industries, and agriculture is no exception. These technologies can analyze vast amounts of data from various sources, such as weather patterns, soil conditions, crop health, and historical pest data, to identify patterns and predict future outbreaks. The key components of AI and ML in agriculture include:
1. Data Collection and Integration: Utilizing sensors, drones, satellites, and other IoT devices to gather real-time data.
2. Data Processing and Analysis: Applying machine learning algorithms to process and analyze the data.
3. Predictive Modeling: Developing models that can forecast pest and disease occurrences based on the analyzed data.
4. Decision Support Systems: Creating user-friendly interfaces that provide actionable insights to farmers and agronomists.
Data Collection and Integration
The foundation of AI-driven pest and disease forecasting is robust data collection. Modern agricultural fields are increasingly equipped with IoT devices that continuously monitor environmental conditions. These devices include soil moisture sensors, weather stations, and drones equipped with multispectral cameras. Satellites also provide valuable data on large-scale crop conditions and environmental changes.
By integrating data from these diverse sources, AI systems can obtain a comprehensive understanding of the factors influencing pest and disease dynamics. For example, high humidity and warm temperatures might signal favorable conditions for fungal growth, while specific soil moisture levels could attract certain pests.
Data Processing and Analysis
Once data is collected, it must be processed and analyzed. This is where machine learning algorithms come into play. These algorithms are capable of identifying complex patterns and correlations that might be missed by traditional analytical methods. For instance, a machine learning model can analyze years of weather data and pest population records to predict when and where an outbreak is likely to occur.
One common approach is to use supervised learning, where the model is trained on a labeled dataset containing historical instances of pest infestations and their corresponding environmental conditions. The trained model can then predict future outbreaks based on current and forecasted conditions. Unsupervised learning, such as clustering algorithms, can also be used to identify new patterns in data without predefined labels.
Predictive Modeling
Predictive models are at the heart of AI-based pest and disease forecasting. These models leverage historical data and real-time inputs to forecast the likelihood of pest outbreaks. Advanced models can incorporate various types of data, including:
- Meteorological Data: Temperature, humidity, rainfall, and wind speed.
- Agronomic Data: Crop type, planting dates, and soil conditions.
- Biological Data: Pest and disease life cycles, migration patterns, and population dynamics.
For example, a predictive model might use weather forecasts to predict the optimal conditions for the spread of a specific fungal disease. If the model identifies a high risk of an outbreak, it can alert farmers to take preventive measures, such as applying fungicides or adjusting irrigation practices.
Decision Support Systems
AI-powered decision support systems (DSS) transform complex data and model outputs into actionable insights. These systems present information in a user-friendly manner, often through mobile applications or web platforms. Farmers can receive real-time alerts and recommendations, helping them make informed decisions about pest management.
A field management system that integrates AI and machine learning can offer several advantages:
- Timely Interventions: Early warnings allow for proactive measures, reducing crop damage and economic losses.
- Precision Agriculture: Targeted treatments based on precise predictions minimize the use of pesticides and other chemicals, promoting sustainable farming practices.
- Resource Optimization: Efficient use of resources, such as water and labor, based on accurate forecasts.
Case Studies and Applications
Case Study 1: Predicting Locust Swarms in East Africa
Locust swarms are devastating for crops and can lead to severe food shortages. Traditional methods of predicting locust outbreaks rely on ground observations and historical data, which can be slow and inaccurate. Researchers have developed machine learning models that analyze satellite imagery, weather data, and historical swarm patterns to predict locust movements. These models provide early warnings to affected regions, allowing for timely interventions and reducing the impact on crops.
Case Study 2: Early Detection of Tomato Leaf Blight
Tomato leaf blight, caused by fungal pathogens, can severely affect tomato yields. An AI-based system was developed to monitor tomato fields using drones equipped with multispectral cameras. The system collects high-resolution images and uses convolutional neural networks (CNNs) to detect early signs of the disease. Farmers receive alerts on their mobile devices, enabling them to apply targeted treatments and prevent the spread of the blight.
Application in Precision Agriculture
In precision agriculture, AI and machine learning are used to optimize pest management practices. For example, a field management system can integrate weather forecasts, soil data, and pest monitoring to create precise irrigation schedules. By maintaining optimal soil moisture levels, farmers can reduce the risk of pest infestations that thrive in specific conditions. Additionally, AI algorithms can analyze crop health data to identify stressed areas that may be more susceptible to pests, allowing for targeted interventions.
Challenges and Future Directions
Data Quality and Availability
The accuracy of AI and machine learning models depends on the quality and quantity of data available. In many regions, data collection infrastructure may be limited, affecting the reliability of predictions. Efforts are needed to improve data collection methods and ensure consistent, high-quality inputs.
Model Interpretability
Complex machine learning models, such as deep learning algorithms, can be difficult to interpret. Understanding how a model arrives at a specific prediction is crucial for gaining the trust of farmers and agronomists. Developing interpretable models and visualization tools can help bridge this gap.
Integration with Existing Systems
Integrating AI and machine learning systems with existing field management systems can be challenging. Compatibility issues and the need for user training can hinder adoption. Collaborative efforts between technology developers, agricultural experts, and farmers are essential to ensure seamless integration and usability.
Ethical and Privacy Considerations
The use of AI in agriculture raises ethical and privacy concerns. Farmers may be hesitant to share data due to privacy issues or fear of misuse. Transparent data policies and robust security measures are necessary to address these concerns and build trust.
Future Directions
The future of AI and machine learning in pest and disease forecasting looks promising. Continued advancements in sensor technology, data analytics, and computational power will enhance the accuracy and reliability of predictive models. Collaborative research and development efforts can lead to innovative solutions tailored to specific crops, regions, and pest threats.
Additionally, integrating AI with other emerging technologies, such as blockchain for secure data sharing and augmented reality for real-time field assessments, can further revolutionize pest management practices. Ultimately, the goal is to create a sustainable, resilient agricultural system that can adapt to changing environmental conditions and ensure food security.
Conclusion
Integrating AI and machine learning in pest and disease forecasting represents a significant leap forward in agricultural practices. By leveraging advanced data analytics and predictive modeling, these technologies provide timely, accurate insights that enable proactive pest management and enhance field management systems. While challenges remain, ongoing research and development efforts hold the promise of creating more sustainable and efficient agricultural systems, ensuring the health and productivity of crops in the face of ever-evolving pest and disease threats.
What's Your Reaction?
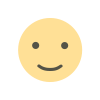
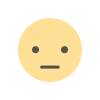
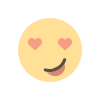
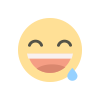
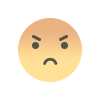
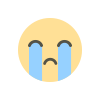
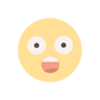